Can post records predict future IPs?
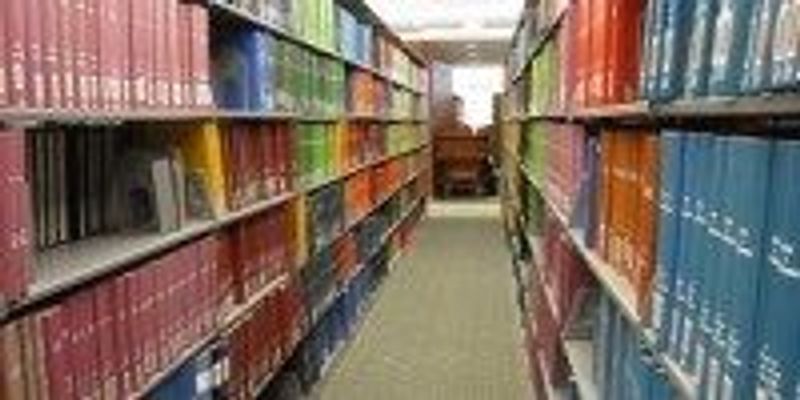
FLICKER, MOON BULBAccording to Lucas Carey from the Pompeu Fabra University of Spain and his colleagues. The team has developed an online tool, called PIPredictor, which uses a machine learning approach to analyze a user’s PubMed data and has already produced more than 800 job success estimates to date. Carey and his colleagues describe their tool in Current biology today (June 2).
“We show that becoming a teacher-researcher is highly predictable, [and] we analyze the characteristics that are predictive” of success, Carey said. The scientist in an email. The algorithm can predict who might become a PI and how long it might take for them to do so with an area under the curve (AUC), a measure of precision, of 0.83 and 0.38, respectively.
While a postdoctoral fellow in Eran Segal’s lab at the…
While having papers in Nature and Science can certainly help, it turns out high-profile publications aren’t the only factors that determine whether an early-career scientist will ever run her own university lab, the team found. Rather, it is the total number of publications, the impact factors of the journals in which they are published, and whether each article meets or exceeds the average number of citations for a given manuscript in that journal that seem most important. In other words, the quantity and quality account. Overall, the researchers noted, more h–indices – measures that attempt to quantify the productivity and impact of a scientist’s publications – are predictive of a greater chance of success in an academic career, supporting a concept first proposed in 2012 by Daniel Acuna of the Rehabilitation Institute of Chicago and his colleagues from Nature.
“However, the gender of the scientist and the rank of their university are also important, suggesting that non-publication characteristics play a statistically significant role in the university recruitment process,” Carey and colleagues wrote in their paper. The researchers found that, given the same publication record and all else being equal, male authors are more likely to become PIs than their female counterparts. Their model controls for both gender and institution rank.
Randall Ribaudo, CEO and co-founder of the professional training company SciPhD.com, spent five years as an PI at the National Cancer Institute’s Immune Cell Biology Laboratory in Bethesda, Maryland, before working in industry. According to PIPredictor, he currently has a 59% chance of becoming a PI, Ribaudo said The scientist. He wondered if the team’s tool could take into account factors such as the academic job market, which has changed significantly over the past few decades. “Over the past 20 years, hiring for tenure-track positions has declined significantly,” he wrote in an email. “Yes [the authors] use longitudinal data and do not account for this downward trend over time, the best statistical probabilities from previous years could result in artificially high numbers.
Paula Stephan, who studies the scientific workforce at Georgia State University, agreed. “Times are changing, and with that, the underlying probability of becoming PI,” she wrote in an email to The scientist.
Stephan added that although the model is “based on a well-constructed bibliometric database”, publication records alone cannot account for “factors that reflect the scientist’s ability to produce the kind of research that can be funded, like innovation [and] creativity.”
Even so, in an increasingly competitive job market, it could be beneficial for a young scientist to get an idea of the status of her publication record. “The tool provides a benchmark that early-career scientists can use to see their relative standing based on publication metrics and university reputation,” Stephan said. “I suspect that many young scientists already have a good idea of where they are, and some a more accurate idea than this. [tool] can provide in their narrowly defined research world.
D. van Dijk et al., “Publishing Parameters and Success in the Academic Labor Market,” Current biology, 24(11), 2014.